We have developed a computational framework for data assimilation of Fraction of Photosynthetically Active Radiation absorbed by vegetation (FPAR) and Leaf Area Index (LAI) from the MODerate Resolution Imaging Spectroradiometer (MODIS) to constrain empirical temperature, light, moisture and structural vegetation parameters in a prognostic phenology model.
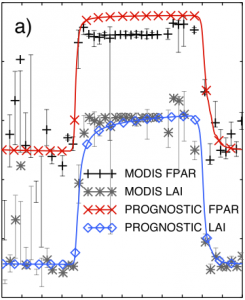
Model-simulated FPAR (red curve) and LAI (blue curve) with a-posteriori parameters after the data assimilation compared to MODIS-based states (grey and black)
It uses the Ensemble Kalman Filter (EnKF) after Evensen [2003] employing around 1000 ensemble members as a sequential data assimilation model to assimilate satellite-derived vegetation states into the phenology model. The data assimilation is very effective in constraining model parameters which then yields a better a-posteriori prediction of vegetation states.
The method is firstly suitable to derive gap-free climatologies minimizing the errors of incomplete satellite retrievals, and it can be applied to parameterize prognostic vegetation models for use in prediction of vegetation states beyond the period of satellite coverage.
All code and related datasets are available as open source to the public:
http://phenoanalysis.sourceforge.net
R. Stockli, T. Rutishauser, D. Dragoni, J. O’Keefe, P. E. Thornton, M. Jolly, L. Lu, and A. S. Denning. Remote sens- ing data assimilation for a prognostic phenology model. Journal of Geophysical Research-Biogeosciences, 113(G4), Nov. 2008. doi: 10.1029/2008JG000781.